Exploring Data Driven Decision Support Systems
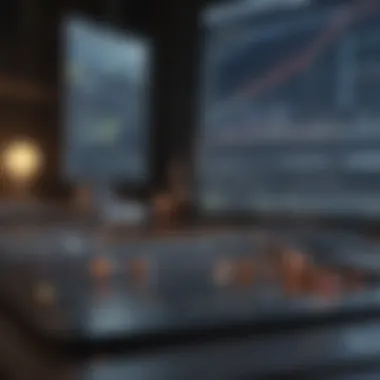
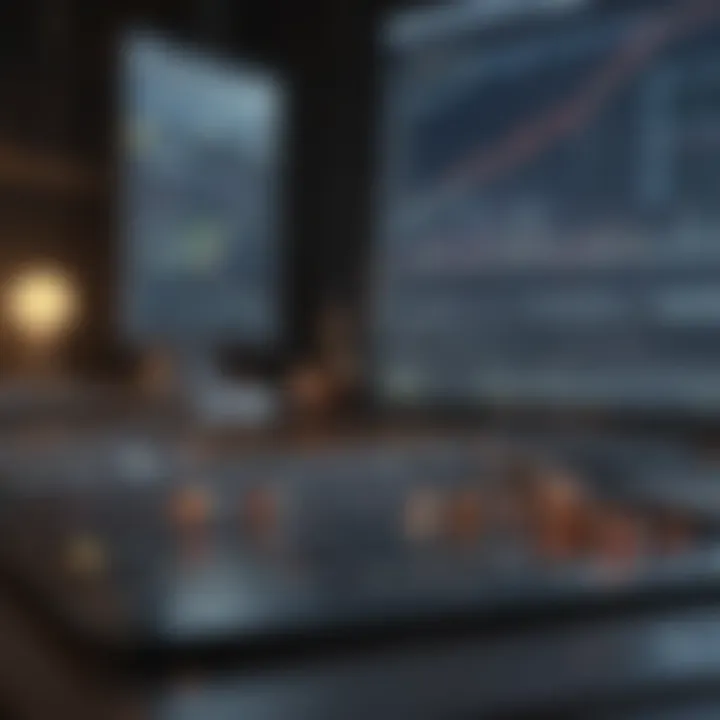
Intro
In today's fast-paced business landscape, making informed choices can feel like navigating a labyrinth with a blindfold on. Enter Data Driven Decision Support Systems (DDSS), a beacon of clarity amidst the confusion. These systems harness the power of data analytics and machine learning to deliver tailored insights, helping decision-makers sift through the noise and hone in on what truly matters.
The significance of DDSS cannot be overstatedβacross various industries, from healthcare to finance, organizations are leveraging these tools to bolster their decision-making processes. This article will unpack the intricacies of DDSS, laying bare the technologies that underpin them, the advantages they offer, and the challenges they present. With a focus on practical applications, weβll also explore different DDSS models and highlight future trends poised to shape their evolution.
Getting a handle on how these systems operate and what they can achieve is crucial for financial enthusiasts and individual investors alike. As you read on, expect to gain a comprehensive understanding of the topicβone that empowers you to navigate the complexities of modern decision-making with confidence.
Key Concepts
Definition of Data Driven Decision Support Systems
At its core, a Data Driven Decision Support System is a nexus of technology, processes, and people, all dedicated to facilitating decision-making powered by data. Unlike traditional support systems that rely on static reports, DDSS taps into real-time data streams, applying advanced analytics techniques to generate actionable insights.
In more tangible terms, think of a DDSS like a sophisticated GPS. Just as a GPS combines maps, traffic data, and calculation algorithms to help you get from point A to point B efficiently, a DDSS marshals vast quantities of data from various sources, processes it, and presents options that can guide the decision-maker toward a desired outcome.
Importance of Data in Decision-Making
In an era defined by information overload, decisions based on intuition alone can lead one astray. By integrating comprehensive data analytics, DDSS minimizes errors stemming from biases and assumptions. Some are saying data is the new oil, but itβs crucial to refine that oil into something usable; otherwise, itβs just a sticky mess.
Consider the following benefits of utilizing DDSS:
- Informed Choices: Provides evidence-based recommendations, lessening reliance on guesswork.
- Increased Efficiency: Automates data processing, allowing quicker responses to changing conditions.
- Risk Mitigation: Identifies potential pitfalls and assesses outcomes before an irreversible choice is made.
However, while the advantages are compelling, itβs vital to recognize that not every organization is equipped to effectively implement DDSS. Drawing upon insights from both tech and business perspectives can bridge that gap.
Expert Insights
The Lay of the Land in Data Support Systems
Experts frequently advise that integrating DDSS into your organization isn't just about technology; it's about fostering a data-driven culture. Executives need to prioritize hiring skilled data analysts and encourage teams to leverage these systems fully. It's not enough to possess the tool; one must understand how to wield it.
"Adopting a Data Driven Decision Support System is akin to training for a marathon. You donβt just show up to the race day; you have to prepare, practice, and ensure conditions are right."
Tips for Successful Implementation
- Align Stakeholders: Ensure everyone from upper management to ground-level employees understands the objectives.
- Educate & Empower: Training is essential. Equip employees with necessary skills to use DDSS effectively.
- Iterate & Improve: Don't view the DDSS as a one-and-done solution. Continuously evaluate its effectiveness and adjust strategies as needed.
Common Pitfalls to Avoid
Even the most well-meaning implementation can falter. Hereβs a few traps that organizations often stumble into:
- Underestimating Data Quality: Inconsistent or poor-quality data can lead to misleading insights; always ensure data cleansing protocols are up to par.
- Overloading Users with Information: A flood of data can cause paralysis by analysis. Focus on what insights are most critical to key decision makers.
- Neglecting Change Management: Resistance to new processes can derail the best-laid plans. Prepare the team for adjustments.
Closure
Through the lens of DDSS, we see a transformative approach to decision-making that is more precise and less prone to the vagaries of human error. As financial enthusiasts or individual investors explore new territories in their decision processes, understanding the principles underlying DDSS can offer a distinct advantage. The interplay between data, analysis, and technology has never been more critical in steering through complex business environments. As the landscape continues to evolve, remaining attuned to these shifts can pave the way for a more strategic future.
Prolusion to Data Driven Decision Support Systems
The landscape of business decision-making is evolving, and the advent of Data Driven Decision Support Systems (DDSS) stands at the forefront of this transformation. In a world flooded with information, having a structured approach to harnessing data is no longer a luxury but a necessity. These systems empower organizations to pivot from intuition-based decisions to choices grounded in data analysis, thereby amplifying the effectiveness and reliability of outcomes.
Definition of DDSS
Data Driven Decision Support Systems can be simply defined as integrated systems that assist in making decisions by converting raw data into actionable insights. It's about taking heaps of data, whether itβs historical sales figures or customer feedback, and synthesizing it into a form that channels effective decision-making. They typically encompass a combination of databases, user interfaces, and analytical tools that facilitate gathering, processing, and analyzing data.
Historical Context
The roots of decision support systems trace back to the 1960s when computers began to influence business processes. Back then, the focus was primarily on data management without the sophisticated analytics we see today. Over the decades, as technologies evolved, so too did the mindset around data utilization. The 1980s and 90s introduced the concept of business intelligence, paving the way for modern data-driven approaches. With the rise of the internet and later, big data, the contemporary definition of DDSS matured, allowing companies to make precise predictions about future trends based on past observations and current variables.
Relevance in Today's Business Environment
In todayβs hyper-competitive market, companies cannot afford to make decisions in the dark. The importance of DDSS is underscored by their role in enabling businesses to harness vast troves of data efficiently. The ability to analyze customer behavior, market trends, and operational efficiency can make or break an organization. Just think about it: companies like Amazon and Netflix thrive not merely on the quality of their products or services but on their uncanny ability to analyze user data to tailor personalized experiences.
"In a world where decisions can make or break, data is the currency of foresight and foresight is the pillar of success."
With more data at their disposal than ever before, businesses that implement these systems glean insights that help them respond proactively to market changes, optimize operations, and improve customer satisfaction. Investors, financial enthusiasts, and organizations that leverage DDSS are not just swimming with the tideβthey are navigating the waters with a compass, charting their course towards growth.
Components of Data Driven DSS
Understanding the components that make up Data Driven Decision Support Systems (DDSS) is essential for grasping how these systems function and the benefits they bring to organizations. These components interconnect and support each other to create a cohesive system that enables effective decision-making. Key components include data management, analytical tools, and user interface and experience, all of which significantly contribute to the performance and usability of DDSS.
Data Management
Importance of Data Quality
Quality data is often the lifeblood of any effective DDSS. High-quality data underpins every analysis and insight generated within the system. It ensures that the decisions made are based on reliable information, reducing risks associated with mistaken data interpretations or flawed recommendations. A noteworthy characteristic of data quality is its multidimensional nature; it encompasses accuracy, completeness, consistency, and timeliness.
In DDSS, prioritizing data quality is a beneficial choice, as it allows organizations to trust the insights derived from the data. Moreover, implementing robust standards and validation procedures can mitigate the drawbacks associated with poor data quality, such as incorrect predictions or misguided strategies. Thus, focusing on data quality continuously within the system can lead to improved decision outcomes and overall efficiency.
Data Sources and Integration
Effective data sources and integration paths are crucial for ensuring that the DDSS has comprehensive information to work with. Companies often pull data from various internal and external sources, from customer databases to social media analytics. The key characteristic here is the ability of the system to seamlessly integrate disparate data sources, which enhances its analytical capabilities.
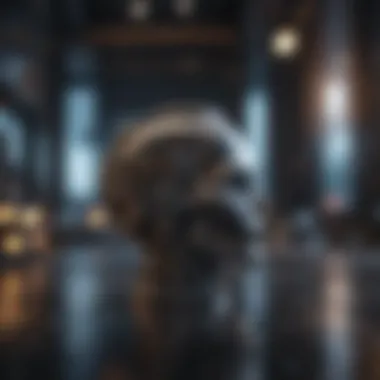
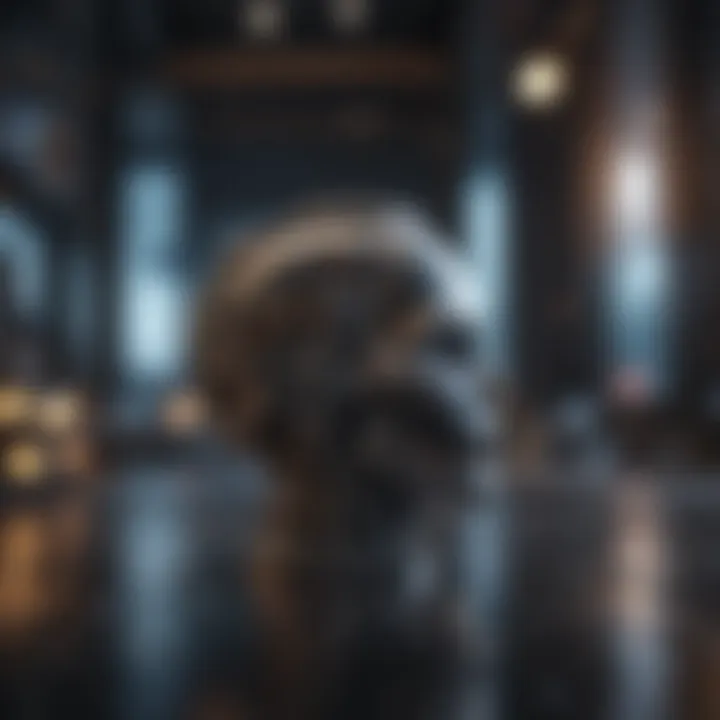
Integration allows for a holistic view of information and supports more nuanced decision-making. This feature, however, can also lead to challenges related to data compatibility and synchronization. Failing to address these integration challenges can result in fragmented data experiences for users and potentially lead to misinformation. Hence, a focus on streamlining data sources and integration is vital to the success of DDSS.
Analytical Tools
Statistics and Predictive Analytics
The backbone of any data-driven system lies in its analytical tools, particularly statistics and predictive analytics. These tools allow systems to identify patterns and trends that inform future scenarios. A significant aspect of these tools is their capability to transform raw data into meaningful insights by utilizing methodologies from statistics and machine learning.
The strength of predictive analytics is its ability to forecast outcomes based on historical data, providing organizations with a competitive edge. However, this advantage can come with limitations such as reliance on the accuracy of historical data and assumptions made during model development. Therefore, while powerful, organizations must approach predictive analytics with an understanding of its context.
Visualization Techniques
Visualization techniques are instrumental in making complex data understandable and actionable. They allow decision-makers to see trends and patterns quickly through graphs, charts, and dashboards. This characteristic not only enhances comprehension but also speeds up the decision-making process by presenting data in a straightforward manner.
One unique feature of visualization techniques is interactivity; users can explore the data at a granular level, which can be significantly advantageous in dissecting information for specific insights. However, it's important to maintain balance in design, as overly complicated visuals can obscure rather than clarify. Therefore, effective use of these techniques in a DDSS must prioritize clarity and user experience.
User Interface and Experience
Design Principles
The user interface (UI) of a DDSS plays a vital role in how effectively users can interact with the system. Good design principles focus on creating intuitive layouts, accessible navigation, and aesthetically pleasing visuals. A crucial aspect of UI design is usability; it should be easy for decision-makers to find the information they need and perform necessary tasks efficiently.
Adhering to sound design principles is not only beneficial for enhancing user satisfaction but also positively affects productivity. However, poor design can lead to confusion and frustration, ultimately resulting in low user engagement. Thus, investing in proper design helps ensure that the tools provided are genuinely supportive of decision-making.
User Accessibility
User accessibility is an essential aspect of the user experience that ensures all users can effectively interact with the system. This includes accommodating different user skills and potential disabilities. Emphasizing accessibility allows organizations to foster an inclusive environment, which is particularly valuable as diverse teams often bring varied perspectives.
A notable characteristic of user accessibility is that it enhances the reach of a DDSS, potentially leading to broader insights and beneficial outcomes. Neglecting this aspect, however, can limit participation and result in versions of the data that do not reflect a full-span view of the issues at hand. Therefore, focusing on accessibility in DDSS design isn't just a nicety; itβs a necessity for fully realizing the potential of data-driven decisions.
"A decision support system is only as good as the data it processes and the design it offers."
Types of Data Driven Decision Support Systems
In the realm of data-driven decision-making, Data Driven Decision Support Systems (DDSS) can be categorized into three vital types: descriptive, predictive, and prescriptive systems. Understanding these distinct types is crucial since each serves a specific purpose and offers unique advantages, making them essential tools for businesses today. The right choice among these systems can significantly impact the quality of decisions made and ultimately drive success in a competitive landscape.
Descriptive Systems
Descriptive systems primarily focus on understanding historical data. They play the role of data interpreters, painting a clear picture of what has happened in the past. This aids organizations in identifying patterns, outliers, and trends, providing valuable insights into their operations.
For instance, a retail store might use a descriptive system to analyze sales data over the past year. This system can highlight which products sold best during certain seasons, helping the business strategize for upcoming trends. Descriptive systems often utilize various data visualization techniques, such as graphs and dashboards, to present their findings clearly. Understanding past performance helps businesses make informed decisions moving forward, making this type of DDSS invaluable.
Predictive Systems
Predictive systems take the baton from descriptive systems and run with it. They analyze historical data and employ statistical methods to forecast future outcomes. By determining the probability of certain events happening, organizations can prepare and strategize more effectively.
Consider a financial services firm using a predictive system to evaluate the likelihood of loan defaults based on past consumer behavior. This system analyzes numerous factors, including credit scores, income levels, and employment stability. With these insights, the firm can make informed decisions about lending policies, ultimately minimizing risk and allocating resources wisely.
Predictive analytics not only allows organizations to identify potential issues but also optimizes decision-making processes by providing a sense of direction based on quantifiable data.
Prescriptive Systems
Finally, prescriptive systems take this a step further. They not only predict what is likely to happen but also recommend specific actions to achieve desired outcomes. These systems utilize optimization and simulation algorithms to suggest the best course of action.
Picture a logistics company that uses a prescriptive system to optimize its delivery routes. By analyzing various factors like traffic patterns, weather conditions, and delivery schedules, the system can recommend the most efficient routes to minimize time and fuel costs. This type of DDSS helps businesses not just understand their situation but actively improves their operational strategies.
"Prescriptive systems transform data into actionable insights, enabling organizations to not only anticipate the future but also shape it."
In summary, understanding the different types of Data Driven Decision Support Systemsβdescriptive, predictive, and prescriptiveβequips decision-makers with the right tools for analysis. Each system plays a pivotal role in transforming raw data into strategic insights, enabling more informed and effective decision-making.
Advantages of Data Driven Decision Support Systems
Data Driven Decision Support Systems, or DDSS, present a range of advantages that shape how organizations approach decision-making. These advantages extend beyond mere automation; they enable businesses to make informed choices grounded in data rather than guesswork. In a world where the only constant is change, these systems allow companies to adapt and thrive. Let's look into them more deeply.
Enhanced Decision Quality
When it comes to making decisions, the difference between a good choice and a great one often hinges on the available information. DDSS brings a treasure trove of data to the table. By utilizing vast sets of data, these systems provide a more rigorous foundation for decisions. Better data means better insights. Businesses no longer rely on instinctual reactions, which, to put it bluntly, can be akin to shooting in the dark.
For instance, when a marketing team considers launching a new product, they can analyze market trends, consumer behavior, and historical sales data. This detailed analysis leads to decisions that are based on concrete evidence rather than hunches. With tools able to apply predictive analytics, the teams can model various scenarios and evaluate potential outcomes, enhancing the overall quality of their decisions.
Increased Efficiency
Efficiency is like the oil in the machine of any organization. DDSS streamlines decision-making processes by organizing and processing data seamlessly. In industries where time is money, this speed can translate to significant savings and competitive advantages.
- Automated Data Processing: Manual data analysis can be both time-consuming and error-prone. DDSS cuts that clutter, allowing for quicker, more reliable insights.
- Real-Time Information Access: With systems designed to pull data from various sources in real time, the decision-makers can act on the freshest information. This is crucial in fast-paced sectors like finance or e-commerce, where the landscape shifts daily.
When companies access insights faster, they can seize opportunities before the competition does. Imagine a retailer who, through a DDSS, identifies a spike in demand for a certain product. They can adjust their supply chain on the fly. Thatβs not just efficiency; thatβs leveraging data as a strategic asset.
Informed Risk Management
Understanding risk is crucial in todayβs business landscape. DDSS provides a structured approach to risk evaluation. By analyzing data patterns, these systems help organizations identify potential risks before they become full-blown crises.
For example, in the financial sector, institutions can use DDSS to track market fluctuations and manage portfolio risks. As trends change, these systems can alert managers to potential downturns or bubbles.
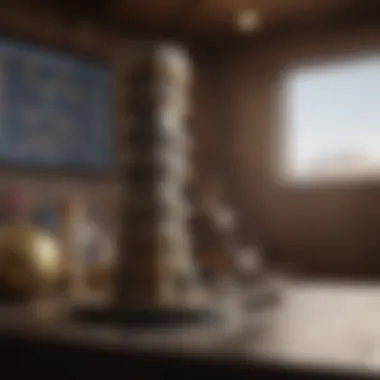
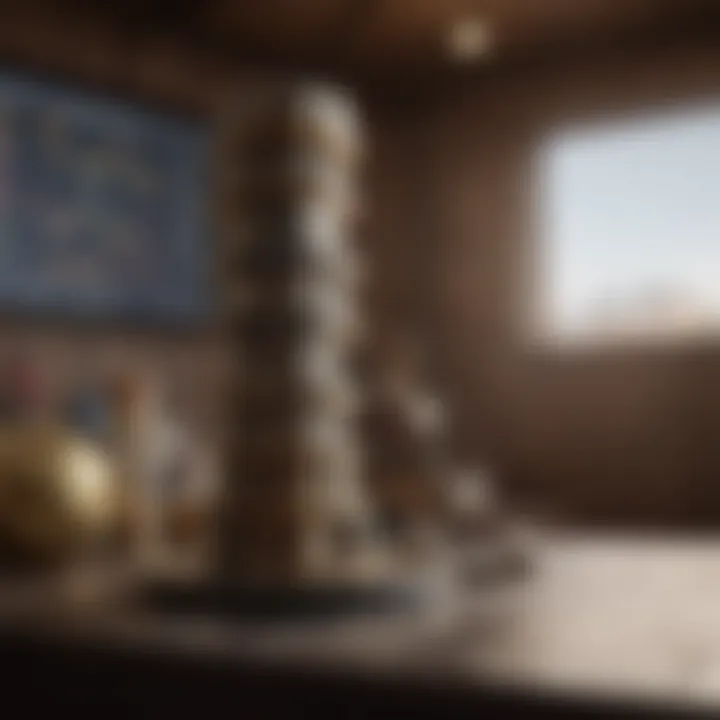
βData-driven strategies arenβt just about maximizing profits; theyβre also about minimizing losses.β
With informed insights that spotlight risks, businesses can develop contingency plans or alter strategies proactively. This foresight transforms risk into opportunity.
In summary, DDSS not only enhances decision quality, boosts efficiency, and refines risk management but also repositions organizations for long-term success. By embracing data-driven approaches, businesses navigate complexities with confidence and agility. The advantages of adopting these systems aren't merely incremental; they represent a paradigm shift in strategic decision-making.
Challenges in Implementing Data Driven DSS
Implementing Data Driven Decision Support Systems (DDSS) presents a multitude of challenges that organizations cannot afford to overlook. Recognizing these obstacles is crucial for leaders aiming to harness the full potential of data analytics and supportive technologies. The increasing interdependence of strategic operations on data-driven insights amplifies the need for a keen understanding of these hurdles.
Data Privacy Concerns
Data privacy has become a major hot-button issue in recent years. With growing awareness regarding personal data safety, organizations using DDSS must tread carefully. The sensitive nature of data collectedβfrom customer information to financial recordsβcan leave businesses vulnerable to breaches if not handled with care. According to a survey by IBM, nearly 60% of consumers feel that companies disregard the importance of data privacy.
Implications include:
- Regulatory Compliance: Data protection regulations, like GDPR and CCPA, impose strict rules on how businesses handle personal data. Non-compliance can result in significant fines.
- Trust Issues: Customers become hesitant to share their data if they perceive a risk. Thus, a damaged reputation may lead to lost revenues.
"Protecting data is not just a technical requirement; it's a strategic necessity in retaining customer trust."
Addressing privacy concerns can involve investing in robust encryption methods and establishing rigorous data governance policies, both of which can be time-consuming yet essential for long-term success.
Technical Complexity
The second major hurdle involves the inherent complexity of the technology itself. Integrating a DDSS into existing systems often requires substantial resources and technical know-how. Many organizations face difficulties due to several factors:
- Legacy Systems: Many businesses rely on outdated technology, making integration with modern tools cumbersome.
- Data Interoperability: Connecting disparate data sources presents challenges in ensuring compatibility and real-time data availability.
- Scalability Issues: As a company grows, the DDSS must adapt on the fly, which can strain infrastructure and demand additional resources.
Organizations must allocate sufficient time and budgets to manage these complexities. This could involve hiring specialized personnel or engaging consultants familiar with DDSS technologies.
User Resistance
Lastly, user resistance can halt the implementation of DDSS before it even gets off the ground. Employees may be uneasy about adapting to new systems. Several contributing factors among staff can include:
- Fear of Change: Many people find comfort in routine. The thought of shifting to a data-driven approach can be intimidating, especially for those who have minimal exposure to technology.
- Skill Gaps: If staff are not adequately trained to use the system, they may feel overwhelmed, leading to pushback against adopting the new tools.
- Perceived Threats: Sometimes, employees might view data systems as threats to their jobs, fearing that automation will replace their roles.
To counter this resistance:
- Training and Development: Offering training sessions can help staff become comfortable with new technologies.
- Championing Success Stories: Sharing examples of how DDSS has made positive impacts in the organization can motivate individuals to embrace change.
Recognizing user resistance as a challenge enables organizations to approach the implementation of DDSS thoughtfully, shaping an environment conducive to embracing data-driven changes.
Case Studies of Data Driven Decision Support Systems
Examining real-world applications of Data Driven Decision Support Systems (DDSS) is like peeling an onion. You get a glimpse into how these systems are not just theoretical constructs but practical tools that businesses deploy to gain a competitive edge. As companies navigate their specific industries, they leverage DDSS to refine processes, enhance decision-making, and respond to emerging challenges. Looking into these case studies, we uncover not only successes but also the nuanced challenges faced during implementations.
Healthcare Applications
In the healthcare sector, DDSS plays a pivotal role in improving patient outcomes and streamlining operations. For instance, a prominent hospital in California integrated a comprehensive DDSS, utilizing data analytics to minimize patient wait times. The system drew on historical patient data, which allowed the hospital staff to predict peak times and allocate resources efficiently. This predictive capability meant that patients received care more promptly, leading to higher satisfaction and better treatment outcomes.
Moreover, hospitals use these systems for risk assessment. By analyzing previous cases, healthcare providers can spot trends and make necessary adjustments to treatment plans. For example, if a hospital identifies a spike in respiratory issues during flu season, they can proactively stock up on relevant medications and adjust staffing levels. This level of foresight is invaluable in an environment where every second counts.
"Data-driven insights in healthcare not only enhance operational efficiency but truly make a difference in patients' lives."
Financial Sector Innovations
The financial industry showcases another layer of DDSS application. Consider a well-established investment bank that employed a data-driven decision support system to optimize its trading strategy. By analyzing massive datasetsβincluding historical market trends and real-time financial newsβthe system helped traders make informed decisions, navigating the turbulent waters of stock markets.
This DDSS provided risk assessments based on various scenarios, allowing analysts to visualize potential outcomes. As a result, it safeguarded against unforeseen losses and maximized gains. The integration of machine learning not only improved prediction accuracy but also streamlined typical report generation processes, letting analysts spend more time on strategy rather than mundane tasks.
Retail and Consumer Insights
In the retail world, companies leverage DDSS for understanding consumer behavior on a deeper level. Consider a leading supermarket chain that deployed a sophisticated analytics system to study shopping habits. By capturing and analyzing data from loyalty programs, transaction histories, and even social media engagement, this retailer crafted tailored marketing campaigns.
It employed strategies based on specific customer segments. For example, if data indicated that a significant portion of its customer base favored organic products during the summer, they could ramp up promotions, ensuring those items were prominently displayed. This targeted approach doesn't just elevate customer satisfaction; it also maximizes profit margins.
The Role of Artificial Intelligence in DDSS
In recent years, the integration of artificial intelligence into Data Driven Decision Support Systems (DDSS) has become an essential part to enhance decision-making across various sectors. AI brings a wealth of capabilities that can significantly improve the effectiveness of these systems, allowing organizations to harness data in a more nuanced and strategic manner. From predictive analytics to real-time data interpretation, the influence of artificial intelligence is shaping the future outlook of decision support methodologies.
Machine Learning Integration
Machine learning stands as a cornerstone of AI applications within DDSS, mainly due to its ability to learn from data and make predictions accordingly. Unlike traditional programming, where rules are defined explicitly, machine learning models adapt and become more precise as they process more data. This adaptability not only enhances the quality of insights derived from datasets but also ensures that the DDSS can evolve alongside changing business environments.
For instance, in the financial sector, machine learning algorithms can analyze vast amounts of transaction data to identify patterns indicative of fraud. When illuminated under conventional decision-making frameworks, such scams might easily slip through the cracks. However, integrating machine learning allows organizations to proactively detect anomalies and react swiftly, thereby mitigating risks effectively.
Some key benefits of machine learning integration into DDSS include:
- Predictive Capabilities: Leveraging historical data to forecast future trends.
- Automation of Routine Decisions: Automating simple decision paths to free up human resources for more complex challenges.
- Continuous Learning: As more data flows through the system, models can refine themselves with little to no human intervention.
- Personalization: Tailoring recommendations based on user behavior, which enhances user experience and satisfaction.
"Human analysts can miss essential details in data patterns, whereas machine learning systems can grasp trends and make predictions at unprecedented speeds."
Natural Language Processing Applications
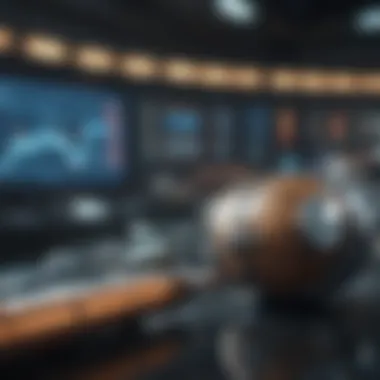
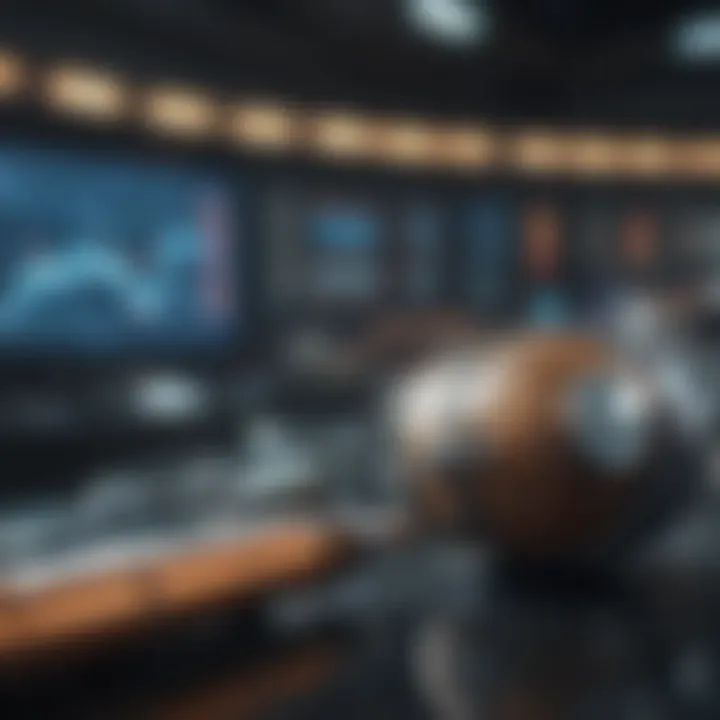
Natural Language Processing (NLP) is another pivotal aspect of incorporating AI into DDSS. It primarily focuses on enabling systems to understand and interpret human language, making it possible for decision-makers to interact with complex data in a more intuitive way.
In practical scenarios, NLP applies to multiple aspects of decision support systems:
- Data Extraction: Automating the extraction of insights from unstructured data sources, such as emails or social media posts, allows organizations to tap into rich veins of information that were previously difficult to analyze.
- Sentiment Analysis: Evaluating customer feedback and social media response can inform businesses about public perception, guiding marketing strategies and product development. Understanding whether the sentiment is positive, negative, or neutral can provide actionable insights.
- Chatbots and Virtual Assistants: Enhancing user interaction helps in facilitating the decision-making process. These tools can answer queries and offer personalized recommendations based on user input, thus combining many data points into a cohesive output.
Ultimately, the integration of machine learning and natural language processing into DDSS stands to arm organizations with the tools necessary to make informed, timely, and effective decisions. As technology evolves, the relevance of these AI applications will only amplify, marking a significant shift in how decisions are influenced by data.
Future Trends in Data Driven Decision Support Systems
The landscape of Data Driven Decision Support Systems (DDSS) is evolving at a rapid pace, and this acceleration is primarily fueled by technological advancements. By acknowledging the future trends in DDSS, we can better understand how these systems will influence decision-making processes across various industries. This part of the article highlights key developments and emerging trends that stand to reshape our approach to harnessing data for strategic decisions.
Evolving Data Technologies
The evolution of data technologies is at the core of the advancements in DDSS. The surge in data generation, coupled with enhanced processing capabilities, is driving innovation like never before.
- Storage Solutions: With technologies like cloud storage, businesses can handle vast amounts of data without the limitations of physical space. This flexibility allows for scalability as organizations grow.
- Data Mining Techniques: As methods become more sophisticated, the ability to extract meaningful patterns from large datasets has vastly improved. Tools are now adept at unearthing insights that can lead to better decision-making.
- Integration of IoT: The Internet of Things is expanding, bringing an array of devices online that generate real-time data. This continuous stream of information provides DDSS with timely insights that were previously unattainable.
Equipping systems with these evolving technologies not only makes them more robust but also helps businesses remain competitive by leveraging the latest data capabilities.
Real-Time Analytics Capabilities
The advent of real-time analytics is a game-changer for decision-makers today. In a world where timing can be everything, the ability to analyze data as it comes in can prevent crises and unlock opportunities.
- Instantaneous Insights: Decision-makers can access live data streams, allowing them to react promptly rather than waiting for traditional reports.
- Operational Efficiency: Businesses are discovering that their operational workflows can be optimized by monitoring performance metrics in real time. This leads to informed adjustments that enhance productivity.
- Boosting Agility: Companies with real-time analytics can pivot quickly in response to market changes, ensuring they stay ahead of competitors.
The benefits of real-time analytics lead to a more proactive rather than reactive strategy, aligning perfectly with the fast-paced nature of today's business environment.
Cross-Industry Applications
The versatility of DDSS extends across various sectors, each finding unique applications tailored to its specific needs. Understanding how different industries leverage data-driven systems can offer insights into best practices and innovative uses.
- Healthcare: Using data analytics, hospitals can streamline operations, predict patient admission rates, and enhance overall patient care. The insights derived can drive significant improvements in both efficiency and quality of service.
- Finance: Financial firms are using DDSS to identify potential investment opportunities, assess risks, and streamline trading strategies. By crunching numbers and analyzing market trends in real-time, they make better-informed choices.
- Retail: This industry benefits immensely from customer data analysis. Trends and purchasing behaviors can guide inventory management, marketing efforts, and customer relationship strategies.
The diverse application of data-driven decision support systems goes to show that no industry is off-limits when it comes to harnessing the power of data.
Integration of Data Driven DSS with Business Intelligence
The fusion of Data Driven Decision Support Systems (DDSS) with Business Intelligence (BI) represents an essential paradigm in the landscape of modern data analytics. It creates a powerful synergy that benefits organizations by enhancing the agility and precision of decision-making processes. This integration is not just about using software tools in tandem; itβs about creating a cohesive infrastructure that leverages data for insightful decision-making. Here we dissect specific elements and advantages that arise from this combining force.
Complementary Technologies
The backbone of effective integration lies in the complementary technologies that support both DDSS and BI. These systems utilize several advanced tools and technologies to manage data effectively. Some critical components that facilitate this integration include:
- Data Warehousing: Both DDSS and BI rely on consolidated data storage solutions that allow users to derive insights from various sources. A well-structured data warehouse ensures faster data retrieval and meaningful analysis.
- ETL Processes: Extraction, Transformation, Loading (ETL) processes are vital as they enable seamless data flow between disparate systems, ensuring that data fed into both DDSS and BI platforms is accurate and consistent.
- Big Data Analytics: As organizations begin to handle larger datasets, employing Big Data techniques allows for more sophisticated analytics. This paves the way for both predictive and prescriptive insights crucial for decision-makers.
- Cloud Computing: The use of cloud technologies allows for greater scalability and accessibility, which is particularly crucial in a rapidly evolving business environment. Organizations can flexibly adjust resources according to their current needs without heavy investments in infrastructure.
The harmony created by these technologies means organizations can scale up their data analysis capabilities, thus providing richer insights for strategically aligned decision-making.
Strategic Synergies
When looking at the bigger picture, the interplay between DDSS and BI fosters several strategic synergies that organizations can exploit:
- Data Quality Improvement: This integration emphasizes high data quality. As BI systems highlight data trends and patterns, DDSS can draw on these insights to refine their data management practices, resulting in cleaner and more reliable datasets.
- Real-Time Insights: By merging the capabilities of DDSS and BI, organizations gain access to real-time insights which significantly accelerate the decision-making process. Decisions can be based on the latest data, leading to timely actions.
- Enhanced User Experience: A unified platform enhances the user experience. Analysts and decision-makers benefit from intuitive interfaces that bring together detailed analytics and decision support functionalities, fostering a more agile decision-making culture.
"The integration of Data Driven DSS and Business Intelligence doesnβt just streamline processes; it transforms how organizations approach and execute their strategic planning and operational decisions."
The collaborative nature of these systems serves to not only enhance the overall effectiveness of decision-making but also reinforces the competitive edge of businesses operating in today's fast-paced market.
Comparison of DDSS Models
When it comes to leveraging Data Driven Decision Support Systems (DDSS), understanding the models available is akin to navigating a sprawling library filled with diverse genres. Each model offers unique insights and methodologies that cater to various organizational needs. A thorough comparison between the different DDSS models sheds light on their distinct functionalities, benefits, and limitations. This comparison not only aids decision-makers in selecting the right system but also enhances the overall effectiveness of their strategic planning efforts.
In today's fast-paced business environment, the choice between different DDSS models isnβt just a matter of preference; it can mean the difference between staying ahead of the curve or lagging behind competitors. By examining aspects such as qualitative versus quantitative approaches and the trade-offs between cloud-based and on-premises solutions, organizations can tailor their systems to their specific contexts with greater precision.
Qualitative vs Quantitative Approaches
Delving into qualitative and quantitative approaches is like choosing between a painter and a statistician. On one hand, qualitative methods focus on subjective insights, often capturing the nuanced elements of decision-making that numbers can obscure. This approach may involve expert opinions, case studies, and anecdotal evidence, helping decision-makers grasp the intricacies of human behavior and complex scenarios. Alternatively, quantitative approaches lean heavily on numerical data, relying on analytics and statistical tools to drive decisions.
Advantages of qualitative approaches:
- Rich context: Provides depth and context that numbers alone can't convey.
- Flexibility: Allows for adaptation and exploration of new ideas based on individual or group experiences.
Benefits of quantitative approaches:
- Objectivity: Minimizes biases through measurable data.
- Scalability: Large datasets can yield broader insights and allow for more extensive predictive analytics.
Ultimately, leveraging both methods in tandem can offer a more holistic view, combining the depth of qualitative analysis with the rigor of quantitative data.
Cloud-Based Systems vs On-Premises Solutions
The debate between cloud-based systems and on-premises solutions is a defining feature of the decision-making landscape for many businesses. Cloud-based solutions, such as Microsoft Azure or Google Cloud, offer scalability and accessibility, allowing users to access systems from anywhere, anytime. This flexibility is particularly beneficial for organizations with remote teams or those that require robust data sharing and collaboration options.
In contrast, on-premises solutions, like those offered by IBM or SAP, typically provide greater control and customization. These systems can integrate seamlessly with existing infrastructures, catering to specific organizational requirements, albeit at the cost of added maintenance and IT overhead.
Considerations when choosing:
- Cost: Cloud solutions often favor pay-as-you-go models while on-premises may involve hefty upfront expenses.
- Security: On-premises systems provide localized security controls, while cloud solutions depend on the provider's protocols.
Both environments come with their strengths and weaknesses, and the right choice hinges on a company's specific operational needs and preferences.
In making a choice between DDSS models, it's essential to evaluate not just current needs but also future scalability and adaptability to changing market demands.